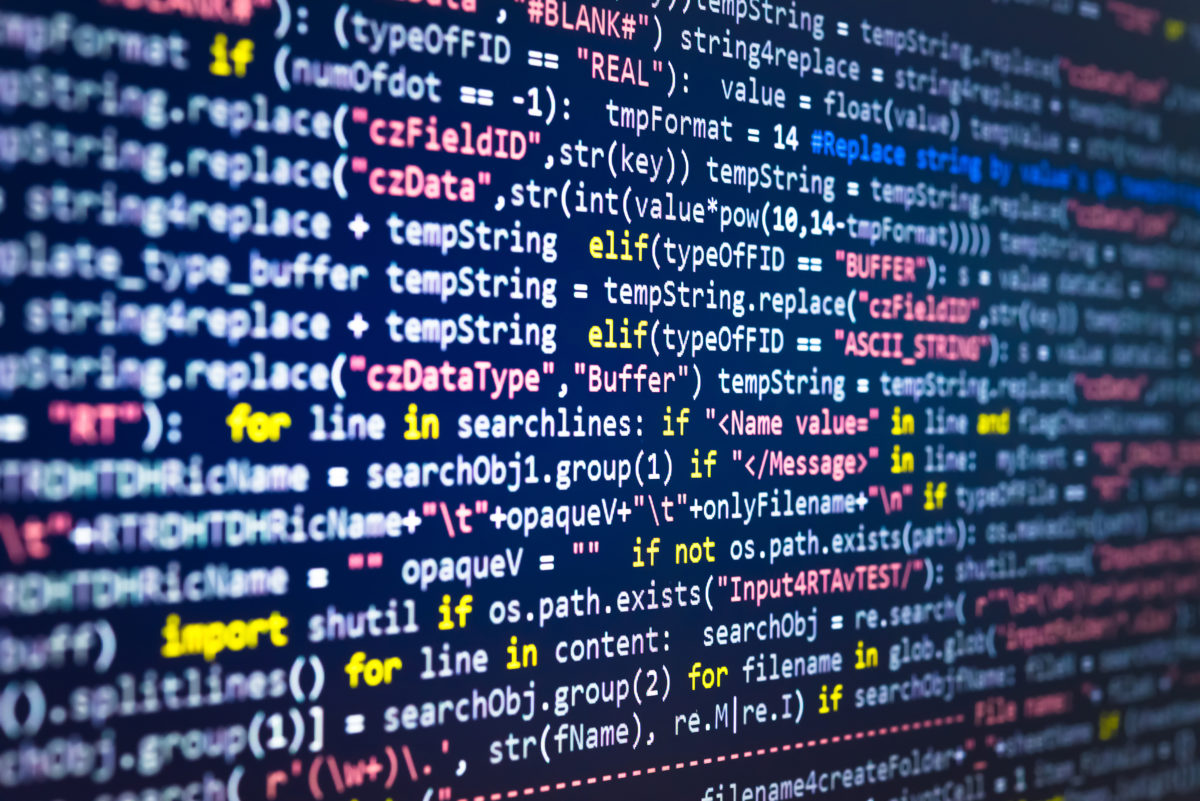
A Beginner’s Guide to AI
From chess playing robots to home automation devices we are increasingly surrounded by artificial intelligence as the seemingly unstoppable progression of smart machines continues. But as the technology grows into ever more dazzling applications and offers potential to augment so many compliance roles, the buzzwords are not always clear and the phrases sometimes overlap. In this short guide, Radar helps you decipher some of the jargon.
Artificial Intelligence
American scientist John McCarthy (1927-2011) first coined the term “artificial intelligence’’ (AI) in 1956 after inviting an array of language simulation and complexity theory experts to a debate. The Boston scientist is believed to have chosen the name to avoid a clash with another track at the time in the field of “thinking machines” and areas such as cybernetics, automata theory and complex information processing. Most dictionaries today focus on AI as a sub-field of computer science and how machines can imitate human intelligence rather than becoming human themselves. The English Oxford Living Dictionary says: “The theory and development of computer systems able to perform tasks normally requiring human intelligence, such as visual perception, speech recognition, decision-making, and translation between languages.” Finance has long been at the forefront of technological advances, and earlier this year JPMorgan Chase introduced a Contract Intelligence (COiN) platform designed to “analyze legal documents and extract important data points and clauses.” Where manual review of 12,000 annual commercial credit agreements can take up to 360,000 hours, an initial implementation of machine learning technology showed that the same amount of agreements could be reviewed in seconds. The bank said COiN has huge potential, as it explores new ways to implement this powerful tool.
Machine Learning
Machine learning is a sub-field of AI, and is best described as a type of computer science that uses statistical techniques to give computer systems the ability to “learn” with data, without being explicitly programmed. The term was developed in 1959 by US computer game pioneer Arthur Samuel, and evolved from the study of pattern recognition and computational learning theory in artificial intelligence. The algorithms learn and avoid having to be reprogrammed by making data-driven predictions or decisions via models built from sample inputs. Algorithmic trading first emerged in the 1970s, and concerned complex AI systems making extremely fast trading decisions. These systems can make millions of trades in a day, hence the term “high-frequency trading” (HFT), which is considered to be a subset of algorithmic trading. While hedge funds and financial institutions rarely disclose their AI approaches to trading, machine learning plays a crucial role in calibrating trading decisions in real time.
Natural Language Processing
Natural Language Processing (NLP) is another sub-set of AI, and also emerged first in the 1950s. It involves an area of computer science concerned with the interactions between computers and human (natural) languages, allowing computers to analyze, understand and derive meaning from large amounts of natural language data. NLP is used to analyze text, allowing machines to understand how people speak. This human-computer interaction enables real-world applications like automatic text summarization, sentiment analysis, topic extraction, named entity recognition, parts-of speech tagging, relationship extraction, stemming, and more. It is commonly used for text mining, machine translation, and automated question answering; all perfect for financial services purposes whether they are chatbots or for compliance staff scanning emails.
The Future
The future of finance is set to be heavily influenced by emerging artificial intelligence solutions as a wave of regulatory compliance offerings using automation continues to grow. Machine learning and NLP are just two examples of how AI is taking the compliance world. In the next issue of Radar we will look at neural networks, supervised and unsupervised learning, as well as the importance of human interaction and analysis, and a transparent methodology that gives regulators and auditors comfort they are not being presented with a black box.