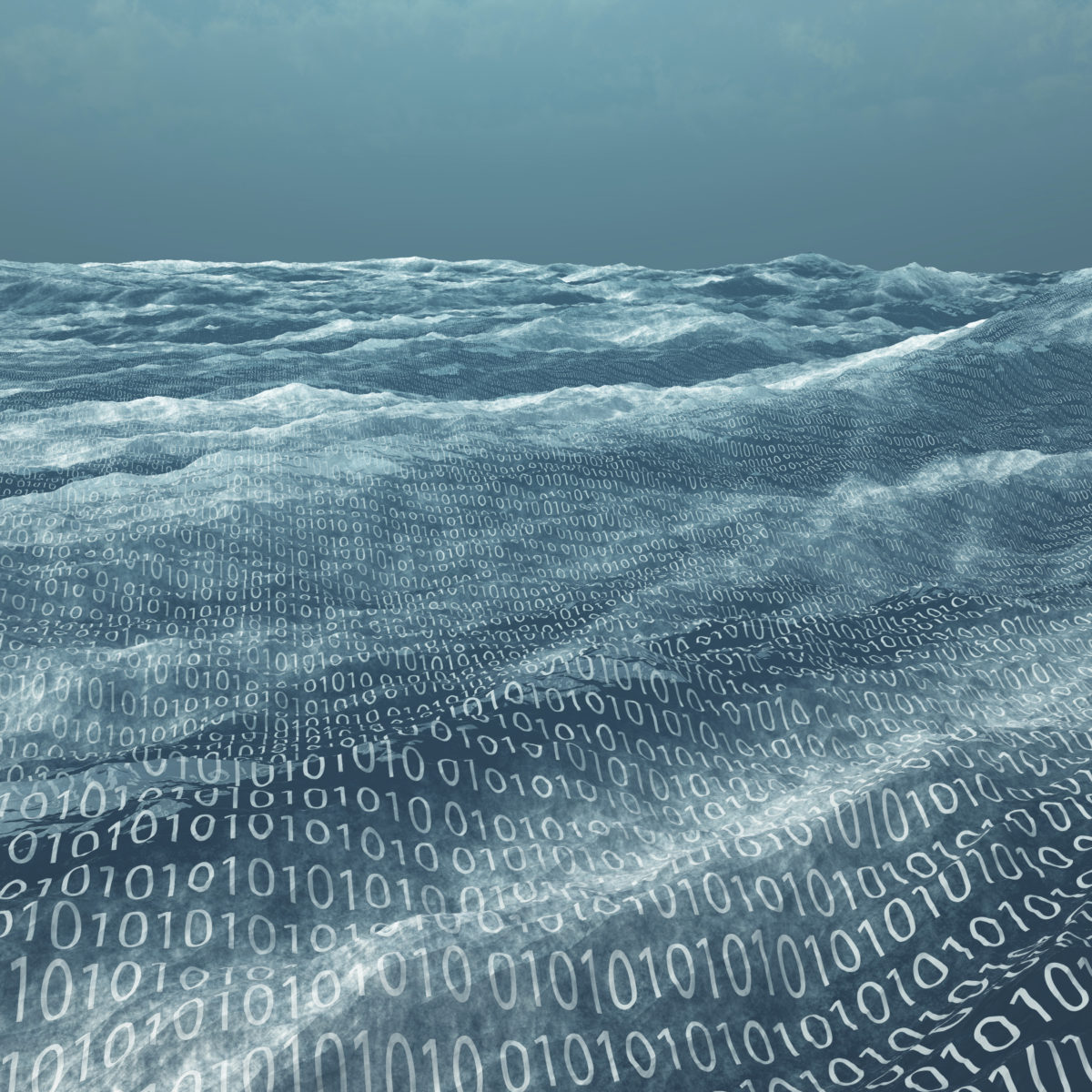
Enterprise Data Lakes – Reality or Pipe Dream?
Big data is as essential to financial services as the internet itself once was, yet so many firms still get cannot get to grips with data management. Radar goes in search of the perfect enterprise data lake.
In a perfect world, an enterprise data lake represents a genuinely centralized marvel of software that contains uniform data and allows the right kind of platform to run programs that give the business the kind of insight that empires are built on.
For many sceptical financial services firms, who have tended to suffer almost two decades of substandard vendor provision that has cost literally millions and resulted in never-ending installations, it has been anything but a perfect world.
Adding insult to injury, the archive vendors have also now infiltrated this expanding market, promising to aggregate everything that a business wants to retain, but offering very limited analytics technology to make sense of the data held.
Data management is becoming the lifeblood of modern business, yet a heady mix of denial, negligence and ignorance is creating considerable challenges across the financial sector, experts told Radar. While not immediately fatal, these experts warn of death by a thousand cuts for those firms trapped in a tightening loop, where their patchwork of different solutions is leading to an outcome that will eventually be impossible to unravel.
“Data is critical in financial services to underpin new products and services, and the reality is most firms are starting from quite a complex state where there are multiple silos due to their many lines of business,” said Nick Millman, managing director, big data and analytics at Accenture Digital consultancy.”
“It may be even more complex if they went through mergers and acquisitions during the financial crisis.”
The concept behind the enterprise data lake is of a single area where data can be consolidated from multiple different sources and analyzed for specific outputs across a number of key functions in the enterprise.
The desired outcome would appear remarkably simple if it can be delivered; a clear storage space that continuously aggregates disparate sources of structured and unstructured data across the enterprise in raw format.
Data ingested is cleaned, normalized, indexed and enriched through processes such as metadata extraction, format conversion, indexing, augmentation, entity extraction, cross-linking and aggregation.
With all the necessary corporate data housed in one repository, advanced analytics can be deployed on top of the lake that can give never-before-seen insights into compliance and risk, culture and conduct, employee performance, customer centric activity, and sales and profit.
Early experimentation in this evolving area has resulted in wasted investments where inferior deployments that have taken several years to install and integrate, have left the vast majority of financial services firms with nothing like a workable solution, said Zhiwei Jiang, global head of insights and data in Capgemini’s financial services practice.
“More than 80 percent of data lakes fail to achieve their ambition. Reason one is most institutions only look at it from an IT point of view; they think as their rivals are doing it and it looks cool, they should too.”
Jiang told Radar that wider business involvement is often less well articulated, despite the fact that any data strategy and implementation by necessity must overlap IT and the business.
“It’s too easy to build a lake now, and firms get overwhelmed,” he said. “You can put so much information in, any kind of data point, it’s easy to input, but harder to get output. This is when the data lake becomes a data swamp. It requires governance, discipline, quality and data monitoring.”
The vogue for data lakes has seen a land grab by a number of compliance archive vendors who have either rebranded themselves as data lake providers or are offering certain solutions with that billing to take advantage of an increasingly lucrative market.
However, what they often neglect to mention is that they are not themselves able to deploy any relevant technology across the top of the data to generate the desired outcomes for the business, which is arguably the most important part. Sort of like owning a car without any petrol to make it run.
The ‘big data revolution’ of the last two decades is the case in point. It has enveloped the banks who have blindly installed systems that captured anything and everything related to data, without any strategic plan that prioritizes connectivity, uniformity, access and interoperability. No clue as to the data required by each function or entity, the types of data set, the end consumer.
“It is the case that many firms are operating flawed data lakes without realizing the lakes are failures,” added Dave Wells, a veteran data analyst at Eckerson Group.
“They build it, carry on feeding data into it, but a miniscule amount of that data is used to provide some value. That is a fairly common scenario for the early adopters, drawn in by the hyperbole, but now they have to go back and rebuild.”
Firms are waking up to the fact that they have multiple data silos spawned from their numerous use of point solutions. Enterprise resource planning, customer relationship management, enterprise data warehousing, cloud and on-premises applications have often been deployed against, or on top of, each other. The result is a congealed mass of inextricable data; a primordial soup that no one wants to own, no one feels a responsibility to fix.
“In the early days of data lakes it was just a place to dump data, and there was not a lot of planning or organization behind it,” said Wells. “As new things have come along, many firms have tried to patch new things on to their old architecture, which they find later doesn’t work.”
Historically most financial services data architectures were structured to handle transactional, structured data. But the exponential growth of retained data is soaring to the extent that there is generally not enough external hardware in existence to hold it.
A typical example might comprise: HR data, sanction lists, restricted lists, gifts and entertainments ledgers, printer logs, call logs, web browser history, corporate calendar, CRM data, revenue and trade data. This is just the start point; then you can add unstructured data such as email, short text messages, chats, voice and social media data into the mix.
Across the enterprise, the often-adopted corporate mantra of “save everything, just in case” has led to the creation of a category called “dark data”, which covers email, instant messages, documents, ZIP files, log files, archived web content, partially developed and then abandoned applications, even code snippets.
Gartner defines dark data as “the information assets organizations collect, process and store during regular business activities, but generally fail to use for other purposes”. It includes all data objects and types that have yet to be analyzed for any business or competitive intelligence, or as an aid in business decision-making.
Operationally effective enterprise data lakes offer the opportunity to make sense of the dark data which is held in significant volumes, but as Millman said, for complex architectures that are prevalent inside a bank, trying to centralize and transform a failed approach can be a daunting, lengthy task.
“We call this a ‘digital decoupling’; in most cases it makes sense to think what your future state data architecture will look like, the data lake will be one key part of that,” Millman said. “It will be a typical place to land most of the organization’s data, and then work out how to consume and process it best.”
The rise of social media has highlighted another key problem, which is the capture of data from outside the enterprise. This is particularly tough because the data will not be clean, uniform, in the right format and comprehensible to the organization from the outset.
“The data quality problem is huge, it’s messy, it’s complex, multi-faceted,” says Wells. “It’s incumbent on anyone using big data that they do proper profiling to judge its quality, and evaluate the veracity of the source, and to make intelligent choices about the use cases.”
For firms looking to get it right, the quality of the data is a hugely important consideration, said Millman. “If you are doing something for regulatory purposes, you will need perfect, or near perfect data and the right lineage back through to understand everything that has happened.”
Another potential headache is data governance, given the level of scrutiny firms now face from the European Union’s General Data Protection Regulation and the ongoing transparency push from financial services regulators such as the US Securities and Exchange Commission.
If the availability, integrity and security of an enterprise data lake is under question, it will make compliance almost impossible, as some big banks have found to their horror. Under GDPR that could mean a fine of more than €20m or four percent of their annual turnover.
“There is a GDPR angle,” said Jiang. “Companies will question if they really want to store everything, and they must really think about their archiving policy. As people become more and more aware of breaches it becomes more tricky.”
If a client leaves a bank, and asks to have their data deleted, banks will question if they can truly achieve this, or if they should instead figure a way to twist and mask, or encrypt it, said Jiang. “That is likely to be a big problem in the future,” he said. “I would not be surprised to see more regulation occur in the data storage space.”
Data governance is one of the key foundations for a successfully deployed enterprise data lake. Firms have also been encouraged to think about what data is required to support specific use cases, concentrating on curation, data cataloguing, and making it easier to digest for the varied users.
A data lake may not be a panacea to everyone, but the right ingredients already exist to create one effectively, if companies are willing to be open to the newer technology, experts agree.
“The data lake of the future is very simple; it will reside in the cloud, and all the bells and whistles will be AI,” said Jiang. “From an ingestion and consumption perspective, it will be so smart; AI in the cloud. It will tell you which data to look at, the machine learning will pick the right data set to pick the right outcome.”