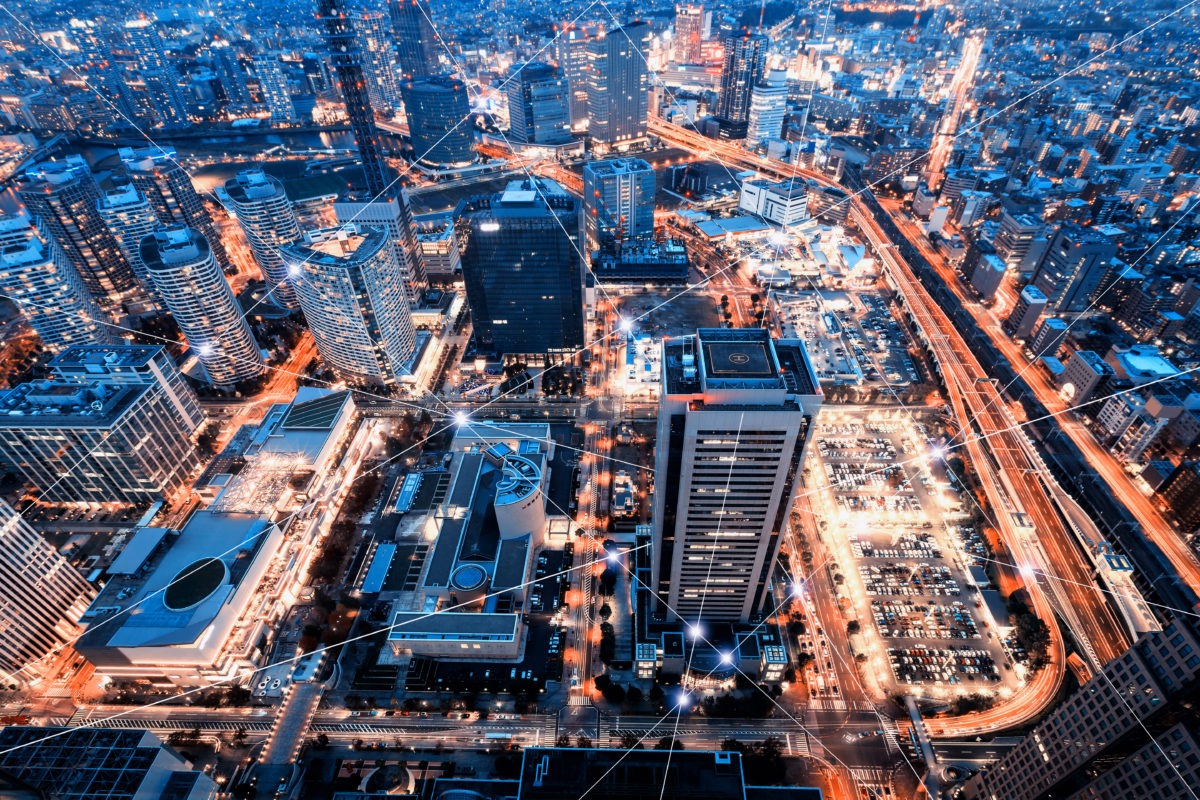
Join the Speech Analytics Revolution and Make Yourself Heard!
With its industry-leading artificial intelligence machine learning engine, Behavox is at the forefront of voice analytics for financial services. Here, senior R&D engineer, Arseniy Gorin, gives his view on some of the cutting-edge projects the company has worked on, and some of the challenges the market faces.
Standard work with voice analytics can be as straightforward as a hedge fund wanting to spot their lexicon of keywords inside their voice files. The Behavox engine transcribes the calls, extracting the textual information and using the lexicon and specific compliance scenarios to generate alerts if there is something suspicious, otherwise known as ‘a hit’, in the call.
More interesting, however, is what we’ve done recently for a large European bank identifying lots of different languages used in phone-calls; English, Portuguese, French, Spanish, Italian to name a few of them. Robustly recognizing language in shorter phone calls is still an open research problem, given the often noisy recordings that are further corrupted by the compression codecs used by the majority of our clients. This is a natural occurrence in most trading environments.
“Yet from about 10 seconds of continuous speech in a call, the Behavox proprietary state-of-the-art neural network-based classifier can spot the language automatically. We can also identify the speaker and link all associated information and communication history.”
While it sounds relatively straightforward, if we can assume a good two-channel recording, with all required metadata associated with the file showing who is calling whom, and their identities, the reality is different.
In practice, properly ingesting and linking the data is a major engineering challenge. What often emerges is a long, continuously recorded single channel signal that can involve up to 20 traders without any information about who is speaking where. Properly formatted and linked metadata is now a high priority task for many of our clients.
Our core technology allows the client to add in a mono audio file, separate the speakers by means of speaker diarization algorithms, and then connect their actual IDs by using voice biometry, or speaker recognition technology. We can train the model to identify the speaker across a much larger data set if we have enough call time stored of decent quality.
Voice quality is still the biggest obstacle to overcome, and this is the main concern for a front office use case we have for a large US bank. They want insights and sentiment extracted from traders’ voices, and the ability to do this is severely impacted by the short length and quality of the calls.
“Trader calls are generally short, and the dialect is unusual (to the outsider); they speak fast, use lots of codes and acronyms. So it becomes more challenging to train models that work well. When referring to a financial instrument, a trader can use a ticker or the full name for a stock, or both.”
Without a protocol, the data becomes more unstructured. This really affects voice transcription, as such an approach requires a rigid vocabulary.
A general-purpose speech transcription engine, such as Google Voice, would simply substitute words it has not encountered before with those it has, and in the world of financial services, with its complex language and endless varieties of company names, this happens all the time. This is a huge problem if stock price tickers are the focus, as it is essential to get this aspect 100 percent correct. The Behavox solution adopts an adaptive vocabulary that allows the addition of new terms both manually and automatically, by mining financial terms from trading and textual data from the client’s data lake.
In terms of the machine learning world, the value is shifting from the already very advanced algorithms to the data, which must be large and well annotated. If we get good quality big data, and we then transcribe it properly, store it, train the good models, that is of huge value to us and our clients.
Many modern commercial voice recorders apply heavy file compression, due to the sheer volume of voice and the lengthy regulatory retention periods, which massively impacts the quality.
“It is often hard enough for a human to understand the calls, let alone a relatively untrained machine. The machine needs a perfect model to be better than a human, and that often requires a very specific task in certain conditions.”
Human operators listening in have more general intelligence than machines, and are better at decoding the voice of a given person in noisy environments, when, for example, several people are simultaneously speaking loudly. This is often referred to as the ‘cocktail party challenge’, as it can confuse machines that pick up some information, but errors are frequent and often too significant for any practical commercial usage. Separating voices in such mixtures without significant quality degradation is yet another unsolved research problem.
In order to improve the robustness of the Behavox acoustic models to noise and other channel degradations, we are using data augmentation, or multi-condition training.
We simulate various compression products, add noises, reverberation, corrupting good data in various manners to make our models better at predicting accurately on highly heterogeneous data that the system may have to work with, including both good and bad quality data. We are adapting our approach to the market conditions, but it would be better for everyone if complete and high-quality data that was well recorded and not compressed was more easily available. The smarter financial institutions are starting to understand this.
“Another big challenge comes from companies requesting multilingual analytics of both textual and spoken communications. Clients want to be able to detect a wide range of languages, transcribe the call, and apply language-agnostic scenarios to that transcription.”
We can do this for English and are gradually adding new languages. The challenge is getting enough transcribed data in the new language with which to train the models.
We are currently focused on collecting and transcribing multilingual data. We are also working on the ability to train our models on limited size data sets that cover obscure or less common languages, where there is not so much available data.
Our English model has been trained on thousands of hours of transcribed phone calls, as an example, but if we want to be able to apply the tech to 80 different languages, the model needs to be able to be trained on less than three hours of data by using joint multilingual neural network architectures and transfer learning state-of-the-art techniques.
If the model is not trained to watch for it, it’s extremely difficult to get accurate multilingual speech recognition and language detection when traders switch languages and mix dialects within a single phone call, which luckily is quite rare.
Apart from linguistic information extraction from voice, clients are also interested in paralinguistics. Finding emotion and sentiment in voice such as when someone is angry, whispering, drunk, or celebrating, are very important areas of focus for clients with the Behavox production-ready solutions.
Paralinguistics for clear recordings, which is also called ‘affective computing’, is making waves in the medical world, where machine learning can help track a person’s emotional state by using various sources of information about the body, including the language the patient uses when talking to a doctor.
From a wider perspective, perhaps from the view of an HR department or senior management, this allows for a better understanding of what is happening inside a company.
Another important aspect to consider is the increased availability of affordable computational power. Clients are more able to deploy large clusters; in the past we have had to compromise with smaller, less accurate models where the client’s compute power is less. The production environment is very different from the research scene.
Another very interesting development is in the area of security and passwords, and the ability to detect if it is someone’s actual voice or a recording of their voice that someone has taken from a voiceprint, to get access to a bank account. This is a different kind of ‘spoofing’ to that of manipulation in financial markets but no less nefarious!
One obvious application from the big tech scene relates to connecting voice analytics with other communication modalities, and Google is a great example.
Users enroll in a large number of services linking them with the same G-suite account, ensuring the analytics platform benefits from real-time linked data derived from user communications, location, behavior in social networks, and so on. It can use these to generate relevant insights (which then might manifest as tailored advertisements to us), or to improve its analytics services.
“The same concept works for most large financial institutions, where a broad range of data are available (textual and voice, trading data, HR data, internal analytics data etc), and unlike anywhere else, at Behavox we do not treat voice as a separate modality, but rather integrate it with all the other data.”
Once engineered properly, the benefits are enormous. It means we can mine patterns, generate insights and behavioral outliers, not only in voice communications, but aggregating multi-modal data across departments, people, or specific projects. Connecting events, or being able to link a phone call about a trade with a preceding email from another colleague with such a suggestion, is a simple example of how linked data can be used in complex company analytics.
We can also improve voice services inside the client environment by using other company communications relevant to the activity. As discussed, most of the machine learning services (especially voice) are difficult to build for every task, condition, or nature of data.
What is really exciting at Behavox is the potential to improve voice services using other communications such as chat logs, which can then enhance speech recognition engine lexicons and language models.
Of course, all of this stays within a client infrastructure, as the security of the data is the paramount concern, making the business of mastering speech in the financial services world tougher than in a B2C platform. It is one of our greatest engineering feats.