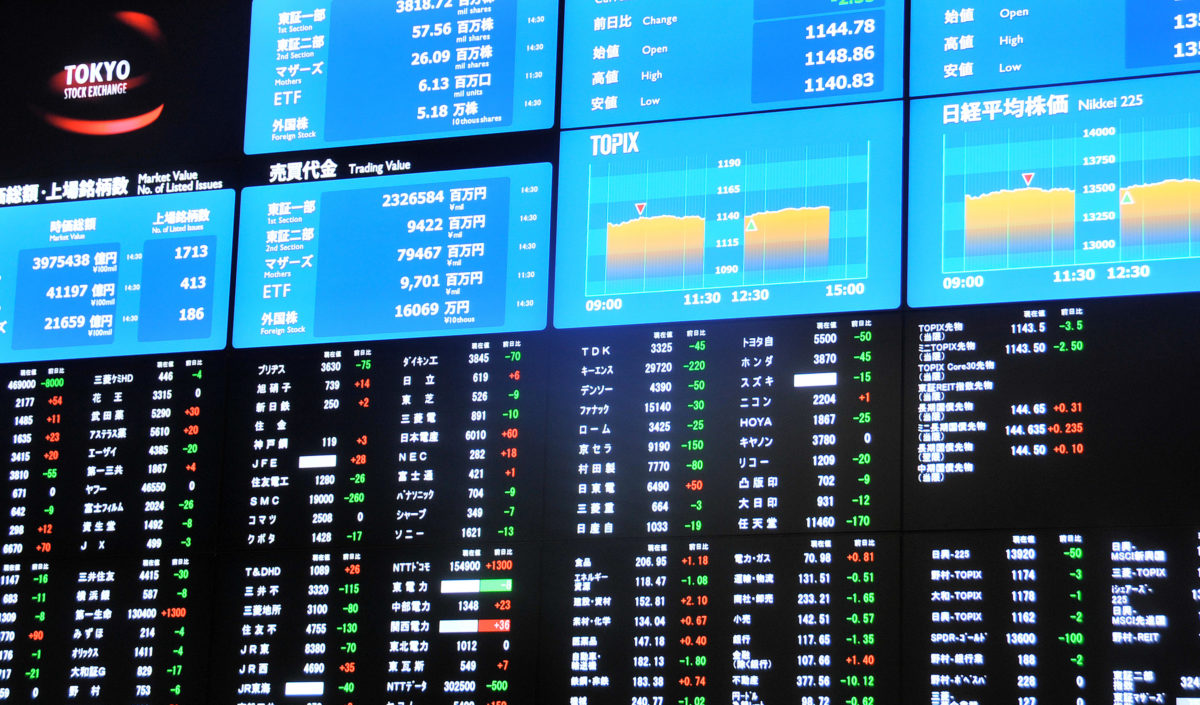
Next Gen Trade Analytics – The Dream and the Possibilities
Radar sits down with a mix of practitioners, ex-regulators and visionary vendors to analyze the potential to turn trade and transaction analytics and surveillance on its head by devising the perfect system to assist the busy monitoring experts in the fast-changing capital markets.
Sit back and close your eyes and imagine the perfect assistant. The transaction-tracking system that seems to know what you’re thinking and delivers data and information to your desktop via a widget in almost real time. A system that means that big question you had about that trade that bubbled into your query queue at market close that was a “hit” but no longer looks like something that needs escalation. Utopia at last. Are we in 2020, 2030 or perhaps 2050?
Trade surveillance and analytics has been established for some time and there is a perception that it therefore should be in a better state than ecomms and certainly acomms. Experts in this area such as Graham Ure, market abuse and surveillance technology partner at PwC, would refute this, stating that trade surveillance is increasingly complex and to do it comprehensively requires a great deal of sophistication in terms of the application of technology, calibration of models, and the procedures designed to support skilled team review of alerts.
Ure says, “there is a misconception that the challenges of trade surveillance have largely been resolved. In our most recent PwC survey we established that somewhere between 70 and 80 percent of all the suspicious transaction order reports (STORs) that get raised are related to insider dealing, not market manipulation. This demonstrates that despite the very sizeable investment in the last two years post- MAR and on surveillance (estimated at £0.75bn among the 21 participants in the recent PwC 2019 Market Abuse Surveillance Survey) that financial institutions are still grappling with the challenge of identifying market manipulation. Or that it does not exist!”
This is not going to be easy
The conclusion is that manipulation is very hard to detect, especially the types that regulators are showing increased interest in, such as intervenue trading and cross-product market manipulation. Practitioners join the chorus and bemoan the silo view they have to contend with when conducting their monitoring and supervision. The head of surveillance at a large market-making broker in New York describes the lack of connectivity between the surveillance team and the systems, “equity, options and fixed income are all overseen by different people and approaches. The current tech does not account for the traders potential to jump across markets and asset classes. The regulators have started to collaborate with each other on this. In the smaller shops the supervisor may cover a number of markets and know the clients and patterns, that is the only hope of joining the dots. But we are all so restricted in terms of time and budget, we deal in quantity not quality right now.”
The limitations of rules-based systems
Radar spoke to six consultants, surveillance heads and vendors in putting this article together and every one stated that the stumbling block here is the use of incumbent rules-based systems. David Hesketh, the founder of TradingHub, puts it logically, “legacy systems were not designed to take advantage of high-compute power. They are essentially augmented relational databases that store trades, orders, positions and market data to enable SQL querying. One of the two incumbent vendors covers all asset classes but fails to understand the complexity of each market. The other has some rule sets and mini-models but is basically the same.
A system needs to think the way the front office does and not just dump it all in a database. It must manipulate that data in a highly mathematical and quantitative way.” A trade surveillance systems architect at another Big Six consulting firm underlined the inflexibility of the current thresholds when failing to account for differences in market conditions and product. He said, “a hit above or below the generic threshold is such a binary way of looking at trading. What matters more is the liquidity and volume of trading in terms of calibration. Generic thresholds here are not going to be accurate signals. This will encourage cute traders to trade happily within certain thresholds but they are still manipulating. What worked 10 years ago is not relevant now.”
It’s the false positives, stupid
Thorough risk assessment of products and sub-products, and subsequent analysis of market abuse risk by asset class viewed through a rules framework delivers an extraordinary number of permutations of potential market abuse to analyse and review. These systems “are then seeking to proverbially boil the ocean, undertaking an exhaustive analysis of the data to eliminate the possible in search of the probable. Put simply, any rules-based system is going to be severely challenged,” claims Ure. This is reflected in the number of alerts generated and was confirmed in PwC’s recent survey. The 21 banks in the survey generated 40m alerts (across trade and ecomms) in a year. STORs resulted from just 0.01 percent of those. The remainder (99.9 percent) were deemed to be false positives. Ure concludes depressingly that “a huge amount of effort is being expended reviewing benign trading behavior and this is inherently inefficient.”
Connect the dots
Talking to the people sitting at the wheel, driving the vehicle and trying to observe the changing laws and conditions of the road about the car of the future that is more robust and current is fascinating in terms of their wishlists. The market-making surveillance head talks about an overlay of data in related markets, connected to the latest media and social posts around a company, as well as earnings and press releases, he says “so much of what I have to clear are exceptions where the system cannot calibrate to the market conditions. I love the idea of a system that can learn but still have the fear it may miss something. I am on the hook for that so it has to be a bullet-proof model.”
All of the practitioners point to the multiplicity of signals now. All can impact a price and behavior or motivation to trade. The surveillance architect likens these to “the sensors for an autonomous car, a sensor on the chassis, the roof and in the engine. All sending messages and allowing the brain to make decisions, navigate, progress. One brain, with one risk model. Currently surveillance is being done by a group of distributed tools with their own risk models, workflow and case management. The need is to bring all this together to create intelligence. Current systems are not designed to communicate, to send data out or to receive instructions. They are passive. Real time information flow for autonomous vehicles is the model.”
Getting rich through gaming
With such a long established approach to trade surveillance and increasingly transparent types of behavior and even parameters used to spot them, is there a real potential for smart market players to game the system and fly under the radar? Everyone we spoke to was pretty confident that the focus on, and transparency within, equities markets left little leeway for manipulation, as one BD trade surveillance head said, “equites has been done to death, and even options is coming into line. But FX and fixed income is the wild west. It’s starting to automate but it’s still like the OTC markets in 80s where you’re making calls, trying to figure out levels. Who can question it if the client is happy, everyone is happy. It’s just not that transparent.”
Those working with “clients” looking to change their ways to adapt to the potential for gaming have interesting war stories. Many of the existing systems only look back five or at most seven days around a shock to reduce the number of false positives. David Hesketh says, “we have had a number of firms say that their traders found out the lookback is seven days and traded abusively 10 days ahead. So they changed it to 14 days. But I then ask them if it might be a concern someone will trade 15 days ahead.”
Risk-based is the only way to go
Once again there was universal agreement on the alternative approach that has to be adopted by the industry and encouraged by the regulators. Graham Ure promotes the cause with some passion, saying “it’s perhaps surprising that the industry has not done more to proactively lobby regulators about the art of the possible.
Hesketh compounds the view, “the industry must move away from lots of rules that need to be calibrated and then approved by the regulator. A risk-based model that works beyond pure transactions and pulls in all the information available (orders, repetition over time etc) and ties it all together into a predictor will deduce what is just noise and what is genuinely interesting. The compliance team then will not be worrying about clearing all the false positives, and will have time to do more fruitful investigation which results in a more cerebral and rewarding role.”
Enabling the next generation
It seems a paradigm shift is required to take advantage of machine learning (ML) and predictive modeling. the challenge for the tech companies is that they need to be met halfway by the clients they aim to service. The machine needs a back book of true positives or “bads” to build the efficacy of the model. Ure suggests that organisations capture and make use of the historic outcomes of their alert review process to generate a richer picture of what their STORs and near misses look like. Once that rationale is stored and codified, it can be used to inform a machine-learning approach leading to the identification of higher risk alerts. ML combined with behavioral and trader profiling has to be the route the industry goes down with the tacit approval or encouragement of the regulators.
Hesketh agrees, “you need to construct a robust model that can account for many different permutations and risks to fit the market parameters in the same way that traders would to evaluate the real price of an instrument or asset. Look at options where the main models devised have been put together by Nobel Prize winners – no one would now price options without a model and using a rule set instead. Why should that be the approach for compliance?”
He continues, “the more complex the trading behavior the more complex the model needs to be, so banging the close, layering and spoofing where you are looking at structure and applying the dynamics of the market, those areas are tough to monitor. There are several orders of magnitude in improvement available from complex statistical analysis.”
Ure concludes, “there is a symbiotic relationship between the technology vendors and the financial institutions; together they can and do drive change. The speed of the change is the key here and the longer it takes the more expense the firms will incur dealing with the inherent inefficiencies we see today. It is surely in the interest of practitioners and regulators to shift away from the perceived imperative of reviewing every alert.”