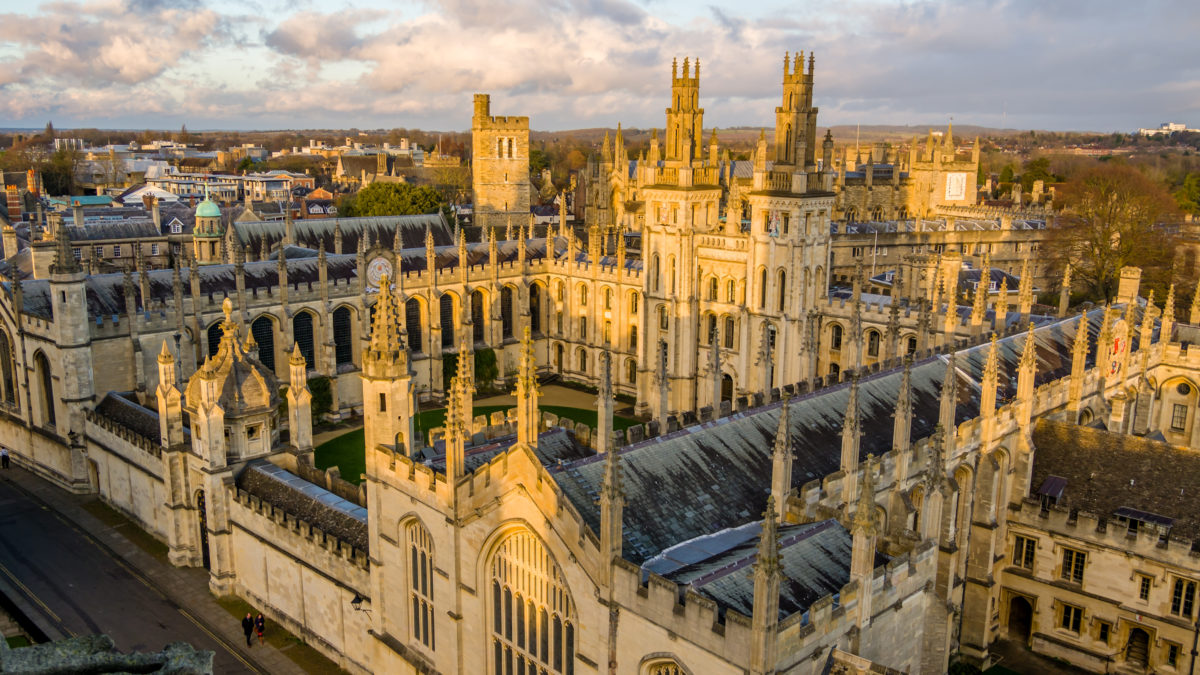
Not So Risky Business: The Academic Expert Using AI to Transform Critical Decision Making
Radar talks to artificial intelligence expert Dr Patrick McSharry of Oxford University about big data, machine learning in finance and politics, and damned lies and statistics.
Few subjects split opinion, and yet are so widely misunderstood, as artificial intelligence (‘AI’). Self-learning robots will either take all our jobs or end the human race, depending on which newspaper you pick up, and yet many who entertain these outlandish scenarios are happy to give commands to devices such as Amazon’s Alexa or Google Home.
What often gets missed are the bits in the middle, where machine learning applied to catastrophes could potentially predict the next financial crisis, or on a local scale where a financial services firm is alerted to employees about to go off the rails that just might prevent the next multi-billion dollar fine.
One man aiming to bust the myths about machine learning is Dr Patrick McSharry, head of the Catastrophe Risk Financing Centre, Smith School of Enterprise and the Environment at Oxford University in the UK.
A celebrated and hugely respected academic with degrees in physics, engineering and mathematics, McSharry has a knack for making complex subjects simple, and while his work exposes him to quantitative approaches, essentially he looks to make sense of the world through science and observation.
The first battle for the Irish-born doctor is dispelling some myths around AI, and convincing people that teaching a machine to do a job traditionally held by a human isn’t the beginning of the end.
“The competition between humans and machines is becoming more intense and this is likely to lead to the loss of many low level jobs that can be automated,” McSharry, who started his PhD in Oxford in 1995, says. “But at the same time, many firms and agencies are still managed by humans with little faith in data or models.”
It is also no surprise to learn some of the world’s biggest investment banks have been knocking at his door for assistance in improving their decision-making and policy choices through advanced analytics techniques.
“I am personally excited about helping the public and private sector to take a data-driven approach to decision-making rather than relying on human judgement,” McSharry says. “This can often involve small amounts of data, but the key is using a rigorous statistical approach to determine when and when not to take action based on the available information.”
Research by IBM shows that more data has been created in the last three years than the previous 5,000 years of humanity, and last year we created more than that in one year alone. However less than 0.5 percent of that data is actually being analyzed for operational decision making.
With more data points to measure than ever, and better tools by which to filter it, financial services firms, traditionally further ahead of the curve in regard to technological innovation than they are given credit for, seemingly have the solution to so many problems in their grasp.
Trade associations say British banks collectively spend £5 billion ($7 billion) annually, and their US counterparts several multiples of that, on financial crime compliance, despite better, faster, cheaper and more advanced solutions to their outdated legacy technology being potentially available.
The threat landscape includes Brexit, ring-fencing, new capital requirements, and increasingly eye-watering fines for misconduct, money laundering and other transgressions, so savings have to be made, and AI will be central to the operations of tomorrow’s financial giants.
McSharry, who lists one of his favourite films as the Dystopian epic Interstellar, is currently working on quantification and financing of risk associated with natural disasters, and explains where machine learning and artificial intelligence enter the picture, and could even help financial regulators.
He uses a catastrophe modelling framework which estimates risk as the product of hazard, exposure and vulnerability, to focus attention on different megatrends that threaten our global economic and financial systems.
“Global supply chains may be more economically efficient most of the time but their fragility and response to shocks from natural disasters have shown how the potential risk is often underestimated,” he says. In 2011, floods in Thailand devastated global electronics and auto supply chains, wiping more than 20 percent off the value of the share prices of Japanese car companies, Toyota and Honda.
Earlier this year, South Africa’s second biggest city, Cape Town, experienced an extreme one-in-384-year drought, which generated losses of US $1.17 billion in the agricultural sector and pushed 50,000 people below the poverty line. “These crises highlight the need for more research, preparation and early warning systems in order to improve responses to extreme events,” McSharry says.
Relationships between hazards and economic losses can be inferred from the analysis of historical extreme events. “Machine learning approaches are capable of uncovering these complicated relationships,” he says.
Applying machine learning to complex relationships goes to the very heart of what some financial firms such as Citibank, RBS, Goldman Sachs and HSBC are doing, either internally or with partners.
“Many organisations are already relying on algorithms [computer code that represents a mathematical model] built using machine learning approaches to detect anomalous behaviour and initiate appropriate responses,” McSharry says.
Compliance and surveillance staff can be trained to create simple algorithms using their own knowledge of what patterns to look for, helping to root out market abuse, sexual harassment, or bribery and corruption inside the data sets that most firms are having trouble controlling.
That is just one of many financial use cases, Dr McSharry says. “Numerous sources of data can be combined to generate credit scores that enable lenders to offer better advice, screen and segment customers and reduce loan default rates. Insurance is becoming more dynamic and tailor-made which means that the insurance premiums can be better aligned with the actual risk,” he says.
His current research concerns the development of quantitative models; combining science and data to generate forecasts for automating decisions and improving policy in areas such as finance, insurance, energy and agriculture.
“With increasing amounts of data about individuals, I am currently working on how to provide insights and decision support to the financial services sector that wish to increase financial inclusion by lending money and innovating the insurance sector. This will build on my research using big data sources such as satellite and mobile call records data analytics to predict poverty levels and estimate the probability of defaulting on loans.”
The proliferation of cheap wireless sensors also makes it easier to observe at a higher spatial resolution, leading to greater forecast accuracy in many areas.
“Intelligence agencies take a similar approach based on the ingestion of multiple sources of information,” McSharry says. “Quantitative hedge funds generate trades each day based on the output of the best model that has been backtested using historical data. Of course humans are still in control but more and more as back seat drivers.”
He says it is important to be balanced and be aware of the potential downfalls of models which are simply caricatures for the real world, however. “Most models will fail if the current situation is no longer similar to the past that has been used to train the model,” he says. “It is therefore important that human oversight is employed with the ability to pull the plug and resort to human judgement when necessary.”
There may be signs that AI is starting to win its public relations war and the resistance is thinning as social and commercial use cases grow. This bears comparison to developments in the blockchain space where decentralized computer network innovations were limited to banks a few years ago but are now being tested as a record filing tool by the National Health Service.
“In many ways, the excitement is greatest in the social sciences where there has been a paradigm shift from the use of focus groups and surveys to accessing data from social media, wearable devices, internet activity and financial transactions to measure the movements, thoughts and actions of large groups of people,” he says.
“The value of alternative sources of data is now being realised and generating discussions about who actually owns the data (mobile call detail records, social media, wearable devices etc),” he says.
This does come with a responsibility for firms, who will be punished heavily for mistakes under the heavyweight EU General Data Protection Regulation which goes live in May. Privacy concerns are undoubtedly high on the agenda, and while in his experience the private sector has always been careful about protecting commercial interests, “the risks for individual citizens are immense”, he warns.
The recent furore regarding Facebook, data analytics firm Cambridge Analytica and suggestions of election tampering as a result of secretive data harvesting are all too close to home, and do untold damage to the reputation of individuals working in the same field, McSharry said.
“The use of the data to target voters with specific messages linked to profiles is at the heart of people analytics. But the repurposing of an academic project to hijack elections is more sinister,” he said. “One thing for sure is that data analytics is going to play a big role in future elections whether we like it or not. Facebook are not alone in holding a treasure trove of information about voters – just imagine if Google were to make profiles available.”
Regulators and governments need to be continually educated about the values of AI innovation, he said, citing the lack of British politicians with science or technology degrees as proof that more lobbying needs to be done in this area, especially given the Brexit-inspired “distrust of experts”.
That the discussion is largely moving on from the scary end of world scenarios to “where else can we pull data from” should however be heartening to innovators inside financial services firms who see artificial intelligence innovation not as science fiction but a reality of modern business.
“Having access to real-time data from multiple sources has the potential to greatly improve decision-making and provide experts with ammunition when attempting to convince policymakers that they can forecast the future,” he says.